Citywide Estimation of Traffic Dynamics Via Sparse GPS Traces
Weizi Li,
Dong Nie,
David Wilkie, and
Ming C. Lin
University of North Carolina at Chapel Hill
Abstract
Perpetual traffic has caused severe congestion in metropolitan areas around the world. The ability to understand traffic dynamics is thus critical to effective traffic control and management. Contrarily, estimation of traffic conditions over a large-scale road network has proven to be a challenging task for two reasons: firstly, traffic conditions are intrinsically stochastic; secondly, the availability and quality of traffic data vary to a great extent.Recent advances in GPS technology and the resulting rich datasets offer new opportunities to improve upon such traditional means. However, such data are limited by their spatial-temporal sparsity in practice. To overcome these issues, we have developed a novel framework to estimate travel times, traversed paths, and missing values over a large-scale road network using sparse GPS traces. Our method enables citywide traffic dynamics estimation, modeling, and analysis.
Discussion
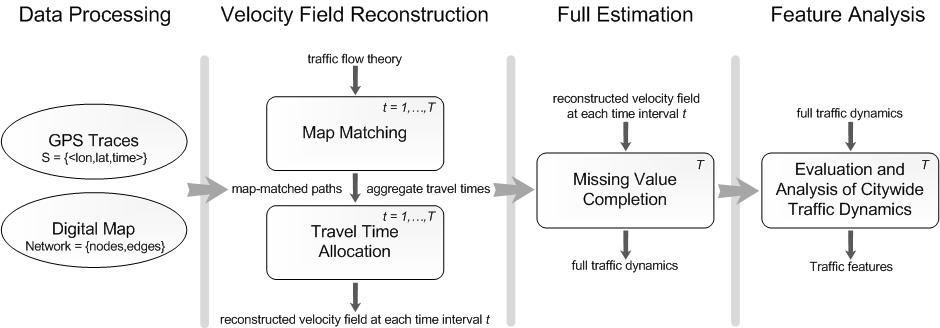
Fig. 1 Overview of the system pipeline. By discretizing one week into hourly intervals, we reconstruct the velocity field in the time intervals where GPS data are abundant. For other time intervals, we estimate missing values to achieve a full estimation so that features of a traffic pattern can be studied.
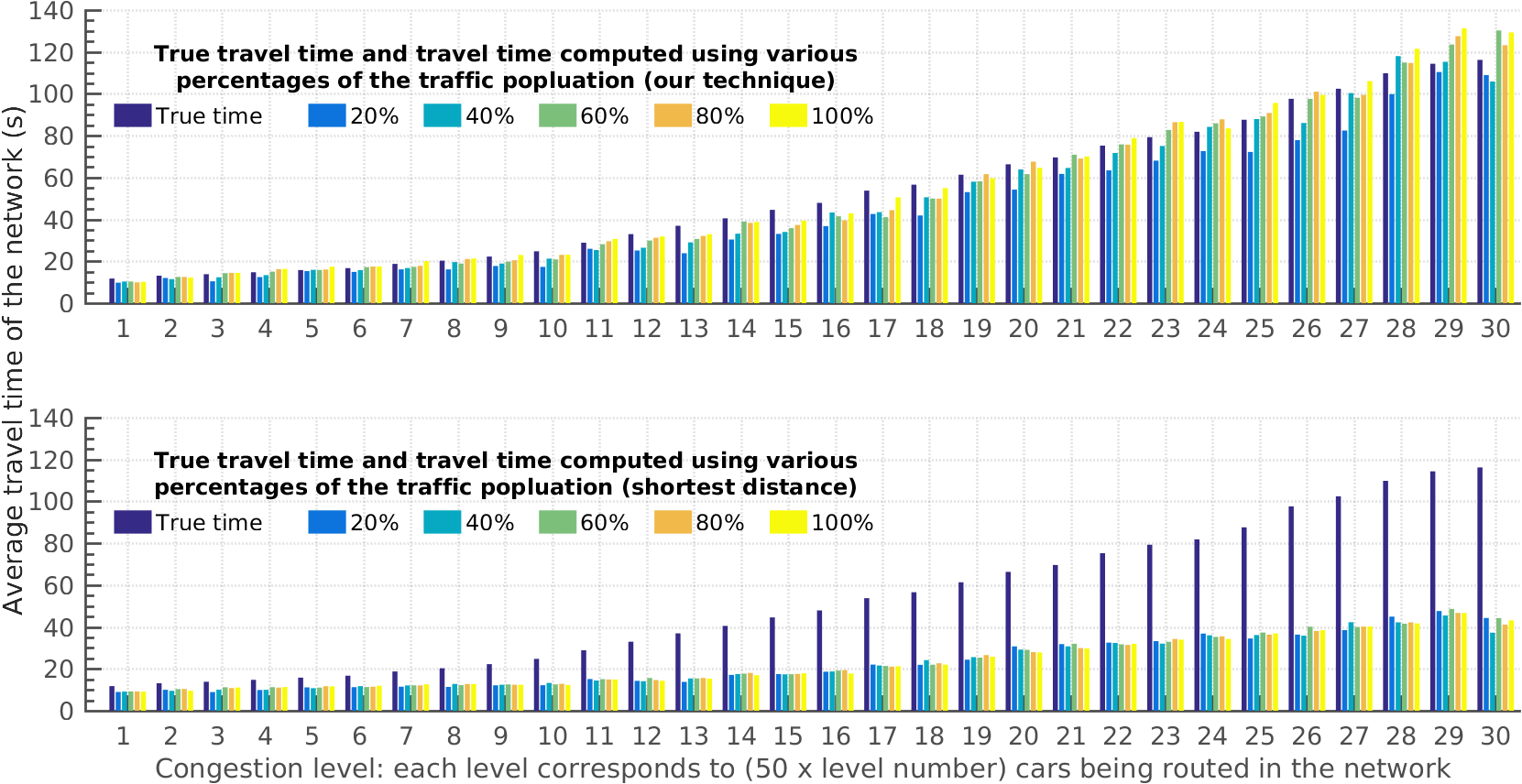
Fig. 2 The reconstructed velocity field results in average travel times on a synthetic road network much closer to the ground truth when compared to the shortest-distance based technique.
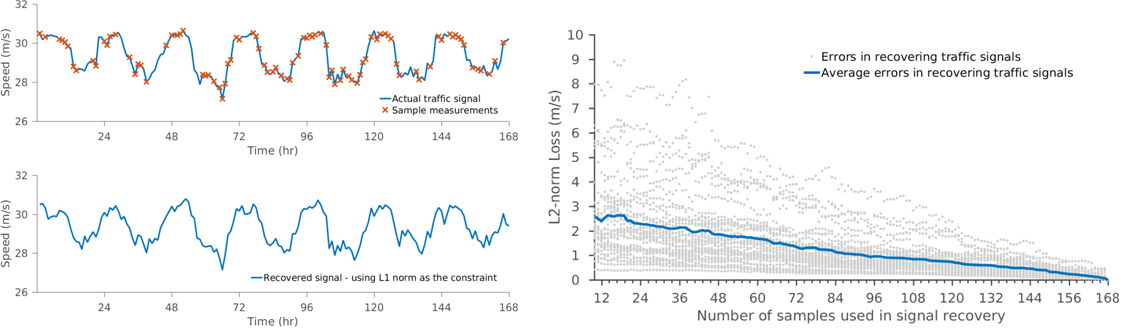
Fig. 3 Traffic signals (i.e., speed readings) over the period of a week are intrinsically periodic. (LEFT) A traffic signal and its random measurements are shown in the top panel. The recovered signal based on random measurements via Compressed Sensing is shown in the bottom panel. (RIGHT) The error between recovered and actual traffic signals. As more samples are used in signal's recovery, smaller errors are observed.
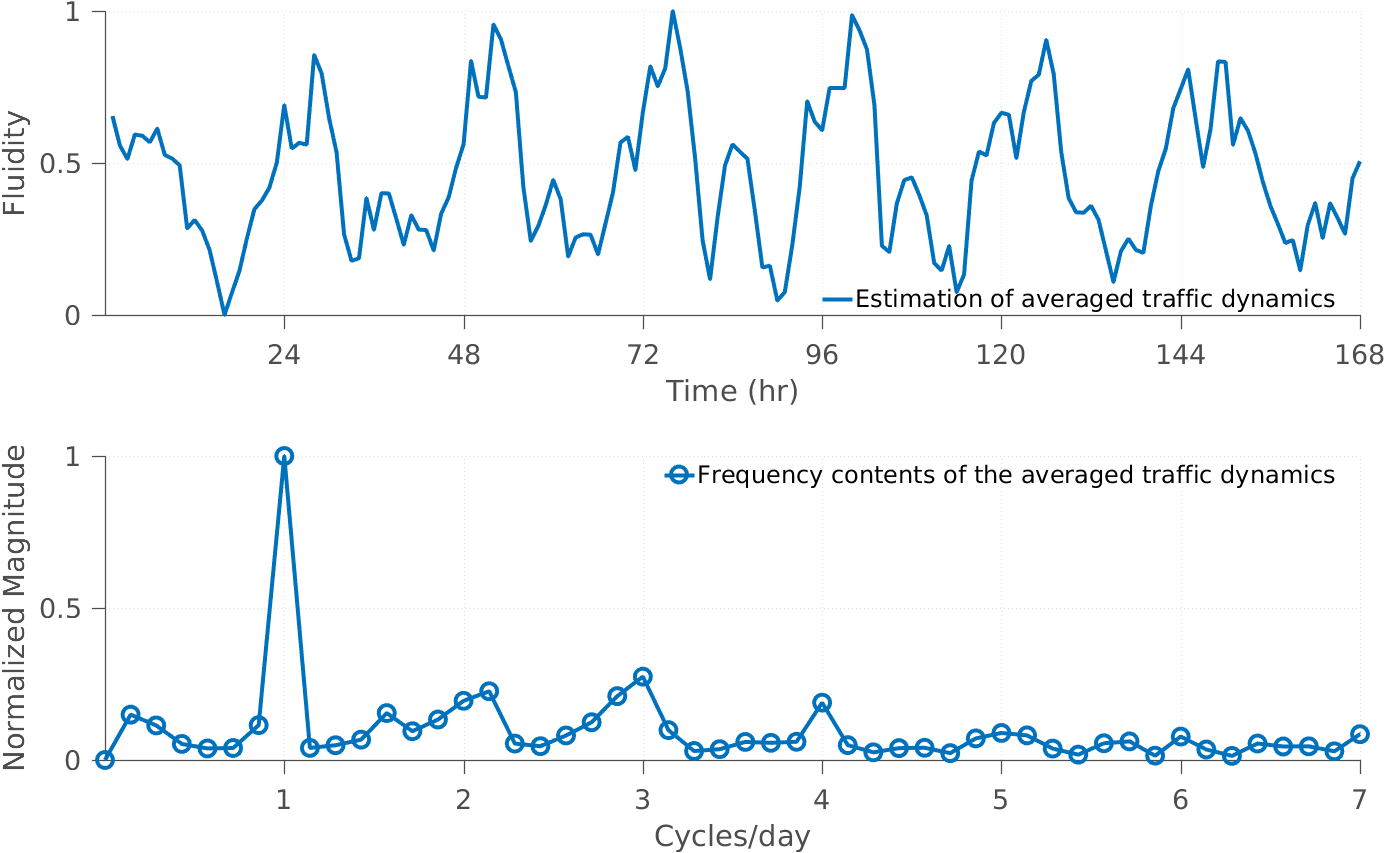
Fig. 4 Estimated traffic dynamics of downtown San Francisco along with the spectral analysis. Our technique recovers the periodic nature of a traffic signal---which is considered as one of the hallmarks of traffic dynamics. This recovery is consistent with the features observed in loop-detector data (which represent relatively accurate measurements) from the same area.
Publication
DOI, IEEE Intelligent Transportation Systems Magazine, 9(3):100-113, 2017Paper (pdf, 4MB)
BibTeX
Acknowledgements
The authors would like to thank National Science Foundation, US Army Research Office, and UNC Arts & Science Foundation.Related Links
GAMMA GroupTraffic Simulation, Reconstruction, and Route Planning